Santiment analysis
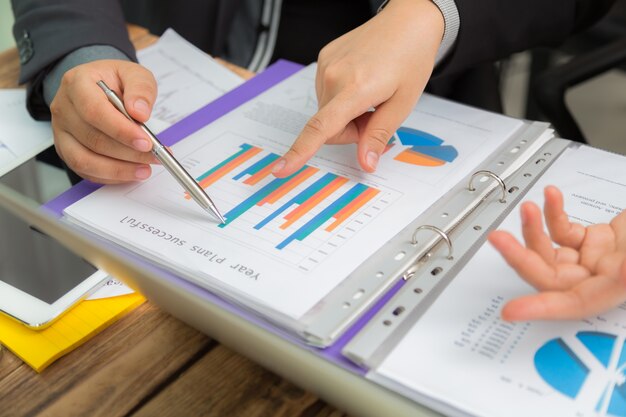
Santiment analysis
In the realm of data-driven decision-making, there exists a formidable force that remains largely untapped. A force that can provide unrivaled depth and nuance to the information we possess, allowing us to make more informed choices and drive progress. It is the art of sentiment analysis – the practice of dissecting and deciphering the emotions, attitudes, and opinions embedded within textual data.
Unleashing the potential of sentiment analysis enables us to uncover invaluable insights that might otherwise remain hidden beneath the surface. By delving into the intricate reservoirs of sentiments expressed by individuals, we gain a profound understanding of their experiences, preferences, and expectations.
Embracing sentiment analysis empowers us to grasp the pulse of the masses, discern the collective sentiment surrounding a specific topic, and identify potential trends and patterns. It equips organizations, businesses, and individuals alike with the ability to comprehend the sentiments of customers, clients, and stakeholders, enabling them to tailor their offerings, campaigns, and strategies accordingly.
By employing sophisticated natural language processing techniques, sentiment analysis allows us to extract meaning not only from positive and negative sentiments but also from the intricacies of sarcasm, irony, and euphemism. This comprehensive approach facilitates decision-making that is not solely based on quantitative data, but also on the qualitative aspects, capturing the essence of human emotion and perception. The fusion of these two realms – the world of hard data and the subtleties of human sentiment – creates a powerful catalyst for innovation and progress.
The Power of Sentiment Analysis: Unlocking Insights for Improved Decision-Making
Exploring the Potential of Sentiment Analysis for Enhanced Decision-Making
In today’s data-driven world, deciphering the sentiments expressed by individuals has become increasingly vital for making informed decisions. A game-changer in this realm is sentiment analysis, a powerful tool that uncovers valuable insights by examining the emotions, opinions, and attitudes conveyed in textual data. By unlocking the potential of sentiment analysis, individuals and organizations can gain a deeper understanding of public sentiment, customer satisfaction, and market trends, ultimately leading to more effective decision-making.
Unveiling Customer Sentiment for Enhanced Business Strategies
The ability to tap into the sentiment of customers is a critical advantage for businesses. Sentiment analysis enables organizations to analyze customer feedback, reviews, and social media posts, revealing the emotions and opinions associated with their products, services, and brand. By comprehending customer sentiment, businesses can identify areas for improvement, enhance customer satisfaction, tailor marketing strategies, and develop new products or services that align with customer preferences. This invaluable insight can drive innovation, foster customer loyalty, and ultimately lead to successful business strategies.
Harnessing Public Sentiment for Informed Decision-making
Beyond the realm of business, sentiment analysis can also be utilized to gauge public sentiment on various social, political, and environmental issues. By analyzing public sentiment expressed on social media, news articles, and online forums, policymakers and opinion leaders can gain valuable insights into public opinion. This knowledge can guide decision-making processes, shape policies that align with public sentiment, and anticipate potential social movements or trends. With the power of sentiment analysis, decision-makers in the public sphere can effectively address public concerns, foster better governance, and bridge gaps between citizens and governing bodies.
Understanding the Influence of Emotions on Decision-Making
Exploring the impact of emotions on the process of decision-making allows us to gain valuable insights into the underlying factors that shape our choices. Emotions play a significant role in shaping our thoughts, perceptions, and ultimately, the decisions we make. In this section, we will delve into the intricate relationship between emotions and decision-making, uncovering how our emotional states influence our judgment and the outcomes we achieve.
The Role of Emotional Intelligence
Emotional intelligence, often referred to as EQ, plays a crucial role in decision-making. EQ encompasses the ability to recognize, understand, and manage our own emotions, as well as the emotions of others. This self-awareness and empathetic understanding of emotions enable us to make more informed decisions. By acknowledging and controlling our emotional responses, we can avoid impulsive or irrational choices, leading to better outcomes.
The Impact of Emotional Biases
Emotions can also lead to biases in decision-making, affecting our ability to think objectively and logically. Positive emotions can create overconfidence, leading to risky choices, while negative emotions can cloud judgment and result in avoidance or indecisiveness. Awareness of these biases helps in mitigating their influence and making more rational decisions.
Types of Emotions | Effect on Decision-Making |
---|---|
Excitement | May lead to impulsive decisions |
Fear | Can result in avoidance or indecision |
Anger | Can create aggression and hasty choices |
Happiness | May lead to overconfidence and risky decisions |
Understanding the influence of emotions on decision-making empowers us to navigate complex choices more effectively. By developing emotional intelligence and recognizing the impact of emotional biases, we can enhance our decision-making processes, ultimately leading to better outcomes and more successful endeavors.
How Sentiment Analysis Helps Identify Trends and Patterns
Sentiment analysis plays a crucial role in uncovering trends and patterns that can greatly impact decision-making processes. By analyzing the sentiment expressed in various data sources, such as social media, customer reviews, and surveys, businesses can gain valuable insights into the attitudes and opinions of their target audience.
Uncovering Emerging Trends
One of the key benefits of sentiment analysis is its ability to identify emerging trends in real-time. By monitoring the sentiment associated with specific topics or products, businesses can quickly identify new interests or preferences among consumers. This allows them to stay ahead of the competition by adapting their strategies and offerings to meet changing consumer demands.
Identifying Patterns in Customer Feedback
Sentiment analysis also helps in identifying patterns within large volumes of customer feedback. By categorizing sentiments into positive, negative, or neutral, businesses can identify common issues or pain points that customers are experiencing. This allows them to prioritize areas for improvement, optimize their products or services, and ultimately enhance customer satisfaction.
- By analyzing sentiment patterns in customer feedback, businesses can identify recurring themes or topics of concern.
- These patterns can provide valuable insights for product development, marketing strategies, or customer service improvements.
- Identifying patterns in customer feedback allows businesses to proactively address issues and maintain a positive brand reputation.
In conclusion, sentiment analysis is a powerful tool that enables businesses to identify trends and patterns within the vast amount of data available. By leveraging these insights, companies can make informed decisions, tailor their offerings to customer preferences, and stay ahead of the competition in an ever-changing market.
Leveraging Social Media Data for Sentiment Analysis
Social media platforms have become a rich source of data for businesses seeking to gain insights into customer sentiment. Analyzing this data can provide valuable information that can inform decision-making and improve business outcomes.
By harnessing the power of social media data, businesses can gain a deeper understanding of how their products or services are perceived by the general public. Sentiment analysis, a technique used to automatically determine the sentiment expressed in a piece of text, allows companies to detect positive, negative, or neutral opinions about their brand or offerings.
Utilizing social media data for sentiment analysis offers several advantages. Firstly, it provides a real-time snapshot of customer opinions and emotions, allowing businesses to quickly respond to any issues or concerns. Secondly, it enables companies to gauge the effectiveness of their marketing campaigns and track the sentiment around specific products or promotions. Lastly, it can help identify trends and patterns in customer sentiment, enabling businesses to make data-driven decisions and stay ahead of the competition.
However, harnessing the power of social media data for sentiment analysis can be challenging due to the sheer volume and unstructured nature of the data. Text preprocessing techniques, such as removing stop words, stemming, and tokenization, are often employed to transform the raw data into a format suitable for analysis.
In conclusion, leveraging social media data for sentiment analysis can provide businesses with valuable insights into customer sentiment, enabling them to make more informed decisions. By analyzing the sentiment expressed on social media platforms, companies can gain a competitive edge and enhance their overall business strategies.
The Role of Natural Language Processing in Sentiment Analysis
Exploring the significance of Natural Language Processing (NLP) in the realm of sentiment analysis brings to light the powerful influence it exerts on discerning emotions, attitudes, and opinions expressed in textual data. When it comes to unlocking insights for informed decision-making, NLP plays a pivotal role in understanding the sentiments underlying human language.
Enhancing Data Interpretation and Classification
Natural Language Processing acts as a catalyst, empowering sentiment analysis by employing computational techniques to comprehend and interpret raw text data. Through the application of linguistic rules, statistical models, and machine learning algorithms, NLP aids in the understanding of sentiment-bearing content, allowing for a more nuanced interpretation of the information.
By leveraging the power of NLP, sentiment analysis can go beyond basic positive or negative sentiment classification, embracing a more granular approach that considers sentiments expressed across a spectrum of emotions. Understanding the range of sentiments facilitates a deeper comprehension of customer feedback, market trends, and public opinion which plays a pivotal role in strategic decision-making.
Uncovering Contextual Dependencies and Tone
Words alone do not always reveal the complete sentiment picture. Contextual dependencies and the associated tone can significantly impact the overall sentiment conveyed. Natural Language Processing algorithms excel at identifying linguistic nuances, metaphorical expressions, and the sentiment intensity embedded within a text.
By delving into the intricacies of language and employing sophisticated techniques like named entity recognition, part-of-speech tagging, and sentiment aspect extraction, NLP empowers sentiment analysis to capture the full meaning behind the words. This level of comprehension enables decision-makers to avoid misinterpretations and make informed choices based on a more accurate understanding of sentiment.
In conclusion, Natural Language Processing plays a vital role in sentiment analysis by enhancing data interpretation and classification, as well as uncovering contextual dependencies and tone. Through its ability to comprehend sentiments in human language, NLP enables decision-makers to gain valuable insights, leading to better decision-making and ultimately, improved outcomes.
Case Studies: Real-Life Examples of Successful Decision-Making Utilizing Sentiment Analysis
In this section, we delve into various real-life scenarios that exemplify the effective utilization of sentiment analysis for decision-making purposes. By leveraging sentiment analysis, businesses and organizations have gained valuable insights into the perceptions, attitudes, and emotions of their target audience or customers.
Through these case studies, we aim to showcase the diverse applications of sentiment analysis across different industries and sectors. We highlight how sentiment analysis has played a pivotal role in assessing customer satisfaction, identifying market trends, enhancing brand reputation, and ultimately guiding strategic decision-making.
The first case study focuses on a retail giant that capitalized on sentiment analysis to gauge customer sentiment towards their products. By analyzing the sentiments expressed in online reviews and social media conversations, the company gained an understanding of the key features that drove positive or negative sentiments. Armed with this knowledge, they successfully improved their product offerings, enhancing customer satisfaction and driving sales.
The second case study revolves around a financial institution that utilized sentiment analysis to monitor the sentiments surrounding their brand. By monitoring social media platforms and online forums, they were able to gauge the public perception of their company and identify potential reputational risks. With these insights, they could proactively address concerns or negative sentiment, bolstering their brand image and maintaining customer loyalty.
The third case study highlights the application of sentiment analysis in the healthcare industry. A medical research organization utilized sentiment analysis to analyze patient feedback and experiences shared on online platforms. By discerning patterns in sentiment, they gained valuable insights into patient satisfaction, as well as areas for improvement in healthcare services and treatments.
These case studies serve as concrete examples of how sentiment analysis has revolutionized decision-making processes across various sectors. By harnessing the power of sentiment analysis, businesses and organizations can make more informed and data-driven decisions, ultimately leading to enhanced success and growth.
Limitations and Ethical Considerations in Sentiment Analysis
Understanding the limitations and ethical considerations in sentiment analysis is crucial for making informed decisions based on the insights derived from this powerful technique. While sentiment analysis provides valuable information about the attitudes and emotions expressed in textual data, it is essential to acknowledge its constraints and ethical implications to ensure unbiased and responsible use.
Limitations of Sentiment Analysis
Despite its effectiveness, sentiment analysis has certain limitations that need to be considered. Firstly, the accuracy of sentiment analysis heavily depends on the quality and relevance of the data used for analysis. Textual data with ambiguous language, sarcasm, or cultural nuances may pose challenges for sentiment analysis algorithms, resulting in inaccurate interpretations.
Additionally, sentiment analysis often fails to capture the complexity and subtlety of human emotions. Emotions are subjective and can vary greatly between individuals, making it difficult for sentiment analysis algorithms to accurately assess the underlying sentiment. A sentiment analysis model may categorize a text as positive, negative, or neutral, but it may overlook the nuances and underlying context that influence the sentiment expressed.
Ethical Considerations in Sentiment Analysis
While sentiment analysis can provide valuable insights, it is important to address the ethical considerations associated with its use. One ethical concern is privacy and data protection. Sentiment analysis relies on analyzing user-generated content, which raises questions about the storage and usage of personal data. It is crucial to ensure that individuals’ privacy rights are respected and that data is handled in accordance with applicable regulations.
Another ethical consideration is the potential for bias in sentiment analysis algorithms. These algorithms are trained on existing data, which may have inherent biases. If the training data is skewed towards a particular group or perspective, the sentiment analysis results may reflect those biases, leading to unfair or misleading conclusions. It is essential to continuously evaluate and mitigate bias in sentiment analysis models to ensure fairness and avoid perpetuating societal biases.
Limitations | Ethical Considerations |
Accuracy depends on data quality and relevance | Ensuring privacy and data protection |
Difficulty capturing complexity and subtlety of human emotions | Mitigating bias in sentiment analysis algorithms |
Q&A: Santiment analysis
What is the primary function of a sentiment analysis tool?
A sentiment analysis tool is designed to analyze text data and determine the sentiment expressed within it, whether it is positive, use sentiment analysis negative, or neutral.
How is a sentiment score calculated within sentiment analysis?
A sentiment score is calculated by assigning numerical values to text data based on the sentiment expressed, aggregating these api values to provide an overall sentiment score for the analyzed content.
What are some common applications of sentiment analysis?
Sentiment analysis is commonly used in market research, customer experience analysis, and social media monitoring to gauge public text analysis opinion and sentiment towards products, services, or brands.
Could you provide examples of positive sentiment identified through sentiment analysis?
Positive sentiment identified through sentiment analysis may include expressions of satisfaction, joy, excitement, or approval machine learning techniques towards a particular subject or topic.
How does sentiment analysis contribute to opinion mining?
Sentiment analysis plays a crucial role in opinion mining by automatically extracting and analyzing opinions, sentiments, dataset and attitudes expressed in text data, facilitating a deeper understanding of public perception.
What role do deep learning techniques play in sentiment analysis?
Deep learning techniques are often employed in sentiment analysis to improve the accuracy and effectiveness of types of sentiment analysis sentiment classification models by enabling the algorithms to learn intricate patterns and representations within text data.
What are some sources of data commonly used in sentiment analysis?
Common sources of data used in sentiment analysis include customer reviews, social media posts, survey responses, online forums, and news articles.
How can sentiment analysis APIs be utilized in real-world applications?
Sentiment analysis APIs provide developers with pre-built tools and functionalities to integrate sentiment analysis capabilities into their applications, enabling automated sentiment analysis of text data.
Could you explain how sentiment analysis contributes to customer experience analysis?
Sentiment analysis helps businesses analyze customer feedback, reviews, and interactions to understand customer sentiment towards their products and services, allowing them to improve customer experiences.
Who typically utilizes sentiment analysis tools and techniques in their work?
Sentiment analysis tools and techniques are commonly used by data scientists, market researchers, social media analysts, and businesses across various industries to gain insights into public sentiment and opinions.
How does sentiment analysis work?
Sentiment analysis involves using natural language processing techniques to analyze text data and determine the sentiment expressed within it, such as positive, negative, or neutral.
What is the significance of sentiment analysis in the modern digital landscape?
Sentiment analysis is important as it helps businesses understand public perception, brand sentiment, and customer opinions towards products or services, aiding in decision-making and improving customer experiences.
What are some common applications of sentiment analysis?
Sentiment analysis is used in various applications such as social media monitoring, brand monitoring, market research, customer feedback analysis, and reputation management.
Could you explain the concept of aspect-based sentiment analysis?
Aspect-based sentiment analysis is a technique that not only identifies overall sentiment but also breaks down text data to analyze sentiment towards specific aspects or features of a product, service, or topic.
How does multilingual sentiment analysis differ from standard sentiment analysis?
Multilingual sentiment analysis extends sentiment analysis to multiple languages, allowing organizations to analyze sentiment across diverse linguistic landscapes and global markets.
What are the challenges associated with sentiment analysis?
Challenges in sentiment analysis include handling sarcasm, irony, and context-specific language, as well as dealing with language nuances, cultural differences, and domain-specific jargon.
How does sentiment analysis use machine learning?
Sentiment analysis leverages machine learning algorithms to train models on labeled datasets, enabling them to automatically classify text data based on sentiment patterns learned from the data.
What role do sentiment lexicons play in sentiment analysis?
Sentiment lexicons are dictionaries or databases containing words and their associated sentiment scores, which are used to assign sentiment to text data during sentiment analysis.
How can sentiment analysis benefit businesses?
Sentiment analysis can help businesses gain insights into customer opinions, market trends, and brand sentiment, allowing them to make data-driven decisions, enhance marketing strategies, and improve overall business performance.
What are some common tools and solutions used for sentiment analysis?
Common tools and solutions for sentiment analysis include sentiment analysis APIs, natural language processing libraries, machine learning frameworks, and custom sentiment analysis software.