Understanding Black Box AI and Its Implications
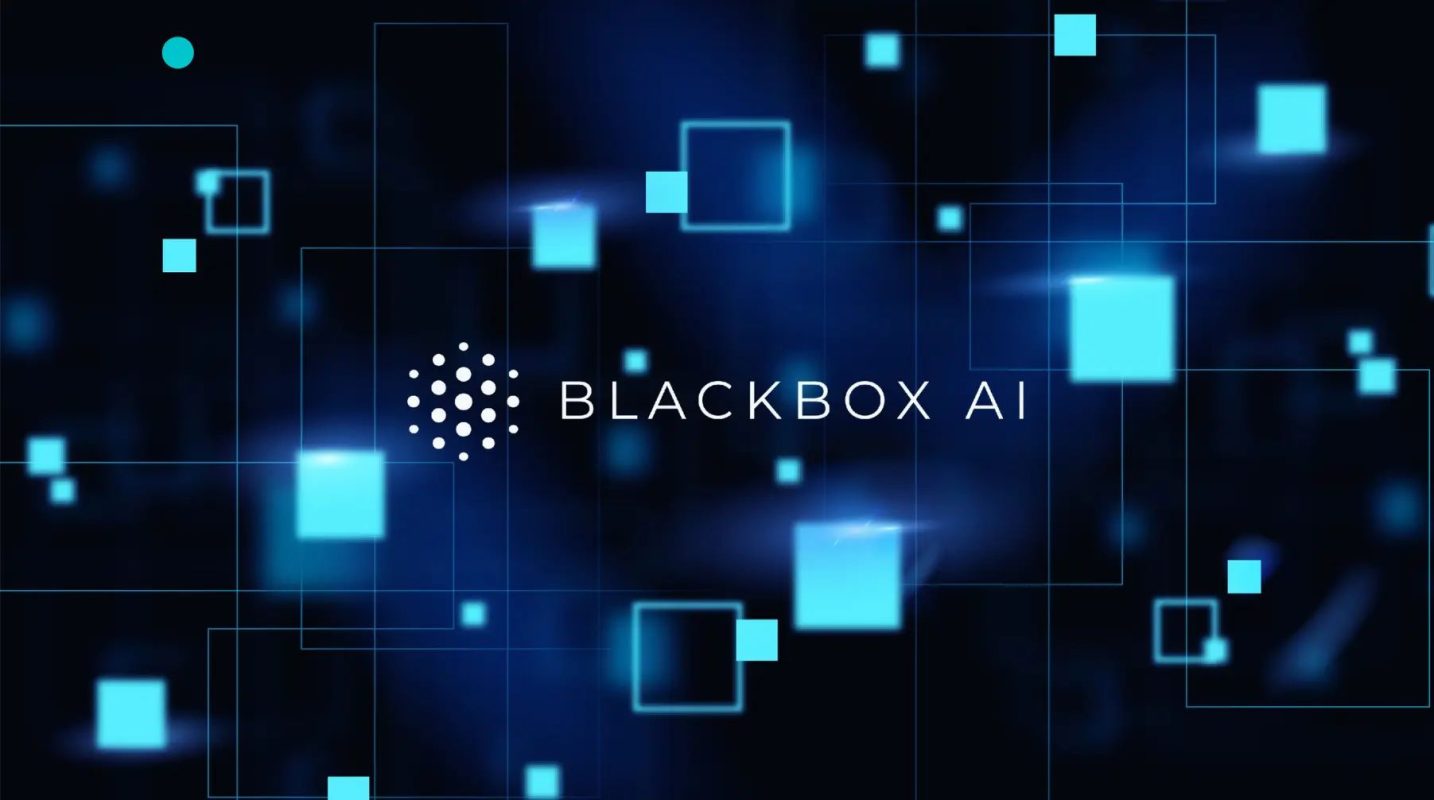
The landscape of modern computing is increasingly dominated by complex systems that operate with remarkable efficiency and seemingly autonomous decision-making. At the heart of these systems lies a profound complexity that often eludes even the most seasoned experts. This complexity raises crucial questions about the foundations of these technologies and the processes driving their operations.
As we delve into this intricate realm, it becomes essential to unravel the layers of abstraction that characterize these advanced systems. Many users engage with these technologies daily, yet few grasp the internal workings that dictate their behavior. This journey seeks to illuminate the subtleties of computation and reasoning that power these remarkable innovations.
In the pursuit of clarity, we will examine how data is transformed into knowledge, exploring the pathways that lead to decisions and predictions made by these systems. A closer look reveals not only the sophistication of algorithms but also the ethical implications entwined with their use and implementation. As we embark on this exploration, the goal is to demystify the core components that underpin these futuristic tools.
What is Black Box AI?
In recent years, certain technologies have emerged that operate in a way that can be puzzling to users and developers alike. These systems often produce results without revealing the rationale or processes that lead to those outcomes. This phenomenon raises questions about transparency, trust, and accountability, especially as reliance on these advanced tools grows across various sectors.
At its core, this type of system is characterized by its complexity, where inputs are transformed into outputs through intricate calculations and algorithms that remain opaque to observers. This lack of clarity can pose challenges for those seeking to understand or predict the behavior of such solutions. As a result, it becomes essential to explore how these systems function and the implications of their usage in critical decision-making scenarios.
While these technologies offer remarkable capabilities, it is vital to navigate the balance between leveraging their power and ensuring ethical considerations are addressed. Stakeholders must remain aware of the risks associated with employing systems whose inner workings are not readily accessible, fostering a dialogue about the need for greater interpretability and oversight in their application.
The Evolution of Artificial Intelligence Design
Over the decades, the framework of computational thinking and problem-solving has undergone significant transformations, shaping the capabilities and applications of machine-driven systems. From simple rule-based applications to complex neural networks, the journey has been marked by innovations that revolutionized how machines learn and adapt to various tasks.
In the early stages, systems were predominantly built on predefined rules and logic. These traditional methodologies relied heavily on human expertise for coding specific instructions. Although this approach proved effective for certain applications, it lacked the ability to generalize beyond predetermined scenarios.
As the field progressed, the inception of machine learning methodologies introduced a paradigm shift. By allowing machines to learn from data, researchers began developing algorithms that could identify patterns and make predictions without exhaustive programming. This approach opened doors to more complex functions and applications across diverse domains.
With the arrival of deep learning, a subset of machine learning, the architecture of systems took another leap forward. Utilizing multi-layered neural networks, these designs enabled machines to process vast amounts of information with remarkable accuracy. This evolution provided significant breakthroughs in tasks such as image and speech recognition, pushing boundaries of what was thought possible.
Today, the quest for improving these computational entities continues. Emerging trends like explainable AI and ethical considerations are steering the design process towards greater transparency and accountability. As we move forward, the ongoing transformation in the architecture and design of these systems promises not only enhanced efficiency but also a more profound understanding of their capabilities and limitations.
Challenges of Transparency in AI Systems
As automated solutions become increasingly prevalent, the necessity for clarity in their operations has gained prominence. Users and stakeholders demand insight into how decisions are made, as a lack of visibility can lead to mistrust and skepticism. Overcoming obstacles related to transparency is essential for fostering acceptance and ensuring ethical practices in the deployment of these technological advancements.
Complexity of Algorithms
One significant barrier to achieving transparency is the intricate nature of various algorithms employed in these systems. Many of these computational models operate on vast datasets, employing complex mathematical frameworks that are not easily interpretable. This complexity often results in a ‘why did it do that?’ scenario, where users are left questioning the rationale behind specific outcomes.
Accountability and Ethical Concerns
Another pressing challenge lies in assigning accountability for the decisions made by these automated systems. As the technology evolves, determining who is responsible for potential biases, errors, or harmful consequences becomes increasingly complicated. This difficulty raises ethical questions about the deployment of solutions that lack clear accountability, further complicating the discourse surrounding their acceptance and use.
Real-World Applications of Black Box Models
Complex algorithms that operate without transparent processes are increasingly influencing various sectors. Their ability to uncover patterns in vast datasets makes them essential tools, although their inner workings often remain elusive. This section explores how these advanced systems are being utilized in different fields and the implications of their applications.
- Healthcare:
In medical diagnostics, opaque models analyze imaging data and patient records to assist in identifying diseases, predicting outcomes, and personalizing treatment plans. - Finance:
Financial institutions deploy intricate algorithms for credit scoring, fraud detection, and investment strategies, providing vital insights while operating beyond straightforward explanation. - Marketing:
Companies leverage these algorithms to analyze consumer behavior, segment markets, and optimize advertising campaigns, aiming for tailored engagement without full clarity on model decisions. - Transportation:
In autonomous vehicle technology, sophisticated systems interpret sensor data to make real-time driving decisions, enhancing safety and efficiency through methods that are not fully transparent to users. - Environment:
Data-driven approaches are used in climate modeling and natural resource management, predicting trends and impacts through complex calculations that may obscure underlying factors.
As reliance on these robust systems grows, discussions around ethical considerations, accountability, and transparency become essential. Understanding the benefits and challenges they present is crucial as innovation continues to reshape various industries.
Ethical Implications of Opaque Algorithms
In today’s digital landscape, the increasing reliance on complex computational systems raises significant moral considerations. As these systems operate in ways that are not always transparent, their effects on society, privacy, and individual rights become a pressing concern. It is crucial to examine the ramifications of utilizing algorithms that function without clear visibility into their operations.
One of the primary ethical dilemmas revolves around accountability. When outcomes produced by these systems lead to adverse results, who is responsible? As developers and companies harness these algorithms, the lack of clarity can obscure lines of responsibility, making it difficult to attribute fault in cases of bias or discrimination.
- Bias and Fairness: Algorithms may inadvertently perpetuate existing societal biases, raising questions about fairness and equality.
- Privacy Concerns: The use of these systems can lead to unauthorized data collection and surveillance, infringing on individual privacy.
- Decision-Making Transparency: Opaque computations can prevent users from understanding how decisions affecting their lives are made.
- Informed Consent: Users may not always be aware that they are interacting with complex algorithms, undermining their ability to provide informed consent.
As society navigates the implications of these advanced systems, fostering a dialogue on transparency, accountability, and ethical standards is imperative. Policymakers, technologists, and ethicists must collaboratively address the complexities associated with the use of algorithms to ensure that advances in technology align with the principles of social justice and respect for individual rights.
Future Trends in Explainable AI
As technology advances, the demand for systems that provide clarity and transparency continues to grow. The shift towards solutions that can elucidate their processes and decisions is becoming increasingly vital across various sectors. This evolution indicates a promising future where interpretability is prioritized, enabling users to not only trust these systems but also to effectively collaborate with them.
One significant trend is the integration of interactivity in explanatory models. Users will be able to engage with algorithms on a deeper level, manipulating inputs to see real-time changes in outputs. This level of engagement fosters a more intuitive understanding of complexities, bridging the gap between sophisticated computations and user comprehension.
Moreover, the incorporation of ethical considerations into these systems is becoming essential. Future advancements will likely emphasize fairness, accountability, and transparency, ensuring that the generated explanations are not only accurate but also just. Developers will need to address biases that may exist within their algorithms and create frameworks to guarantee equitable outcomes.
Additionally, advancements in natural language processing will enhance the ability of these systems to articulate their reasoning. By providing insights in a more relatable and accessible manner, users from various backgrounds will find it easier to grasp intricate processes. This development will make explanations more user-friendly, facilitating wider adoption and trust.
Lastly, collaboration between researchers and industry practitioners is expected to foster innovative solutions. Sharing best practices and insights will accelerate the development of tools that prioritize explainability while maintaining efficiency and performance. As a result, the future landscape will feature a more informed approach to technology that values clarity and understanding as much as functionality.
Q&A: What is black box AI
What does the term “Black Box AI” refer to?
The term “Black Box AI” refers to artificial intelligence systems whose internal workings are not easily understood or interpreted by humans. In these systems, input data is processed to produce outputs, but the specific algorithms and decision-making processes that lead to those outputs remain opaque. This lack of transparency raises concerns about accountability, fairness, and trust, as users and stakeholders cannot ascertain how decisions are being made, especially in critical applications such as healthcare, finance, and criminal justice.
Why is it important to understand the mechanisms behind Black Box AI?
Understanding the mechanisms behind Black Box AI is crucial for several reasons. Firstly, it promotes accountability; stakeholders need to know how decisions are made to ensure fairness and reduce biases in AI outputs. Additionally, understanding these mechanisms can help developers improve the systems, making them more reliable and effective. It also fosters public trust, as users are more likely to engage with AI technologies if they have some assurance about how decisions are reached. Lastly, in regulated industries, understanding AI can help organizations comply with legal standards and ethical guidelines.
What are some common approaches to making Black Box AI systems more transparent?
Several approaches can be taken to enhance the transparency of Black Box AI systems. One common method is the development of explainable AI (XAI) techniques, which aim to provide human-understandable explanations for AI decisions. Techniques such as LIME (Local Interpretable Model-Agnostic Explanations) or SHAP (SHapley Additive exPlanations) help users interpret the outputs of AI models. Another approach is to utilize inherently interpretable models, such as decision trees or linear regression, which are easier to understand than complex neural networks. Additionally, organizations can establish robust auditing and validation processes to ensure that AI systems are functioning as intended and to provide insights into their operational behavior.
What are the ethical implications of Black Box AI?
The ethical implications of Black Box AI are significant and multifaceted. One major concern is bias and discrimination, as these systems can perpetuate or even amplify existing societal biases present in the training data. This can lead to unfair treatment of individuals in critical areas such as hiring, law enforcement, and healthcare. There’s also the issue of accountability; when decisions made by AI systems lead to negative outcomes, it can be challenging to determine responsibility. Furthermore, the opacity of these systems can compromise informed consent, as users may not fully understand how their data is being used or how decisions about them are made. Addressing these ethical implications is essential for the responsible development and deployment of AI technologies.
Are there any specific industries that are more affected by Black Box AI, and how?
Yes, certain industries are particularly affected by Black Box AI due to the critical nature of decision-making involved. In healthcare, for instance, AI systems used for diagnosis or treatment recommendations must be transparent, as decisions can significantly impact patient outcomes. A lack of understanding of how these systems operate can lead to mistrust among patients and healthcare providers. In the finance sector, algorithmic trading and credit scoring systems also face scrutiny, as potential biases can lead to unfair lending practices or financial instability. Similarly, in the criminal justice system, predictive policing algorithms can reinforce racial biases if their inner workings are not scrutinized. These industries emphasize the need for transparency and accountability in AI applications to ensure ethical practices and public trust.
What is “Black Box AI” and why is it a concern in artificial intelligence?
Black Box AI refers to artificial intelligence systems whose internal workings are not visible or understandable to humans. This opacity creates concerns regarding accountability, transparency, and trust, particularly in applications like healthcare or criminal justice, where decisions made by AI can have significant impacts on people’s lives. As these systems often use complex algorithms, even the developers may find it challenging to explain how a specific decision was made. This leads to calls for more interpretable AI methods that can provide insights into how decisions are reached, enabling users to better trust and comprehend the AI’s rationale.
What is the difference between white box AI and black box AI models?
The difference between white box AI and black box AI models lies in their transparency. White box AI is transparent, meaning users and AI developers can understand how an AI algorithm makes its decisions. In contrast, black box AI operates without revealing its internal workings, making it difficult to understand how an AI arrived at its conclusions. This opaqueness of black box AI is often a concern for those focused on responsible AI development.
How do AI researchers address the black box problem in machine learning models?
AI researchers address the black box problem by developing explainable AI or XAI, which focuses on designing AI algorithms that can provide clear explanations for their outputs. This helps users understand how the AI algorithm makes its decisions and how the AI models work. By improving transparency in black box AI, researchers aim to reduce bias in black box AI and increase trust in AI systems.
Why is the use of black box AI models common despite their opaqueness?
The use of black box AI models is common because they can achieve high performance in complex tasks, especially in areas like deep learning. Even though black box AI is used without full transparency, its ability to handle large datasets and complex patterns makes it integral to advancements in AI tools and applications. AI continues to rely on black box machine learning for tasks where accuracy outweighs the need for explainability.
What are the risks associated with using black box AI in decision-making processes?
The risks associated with using black box AI in decision-making processes include the potential for bias in the AI model and a lack of accountability. Since black box AI models work without revealing their decision-making process, users may not know how the AI arrived at its conclusions, leading to errors or unfair outcomes. Addressing the black box problem is essential to ensure AI systems are fair, transparent, and responsible.
How can transparency in black box AI help ensure responsible AI development?
Transparency in black box AI can help ensure responsible AI development by making it easier for AI developers and users to understand how an AI algorithm makes its decisions. This reduces the risk of hidden biases and improves trust in AI systems. By focusing on explainable AI, the future of black box AI may involve more glass box AI approaches, where the internal workings are visible, helping users know how the AI models arrive at their outputs.